Our industry expert and EV evangelist Chris Chamberlain shares four key steps that successful...
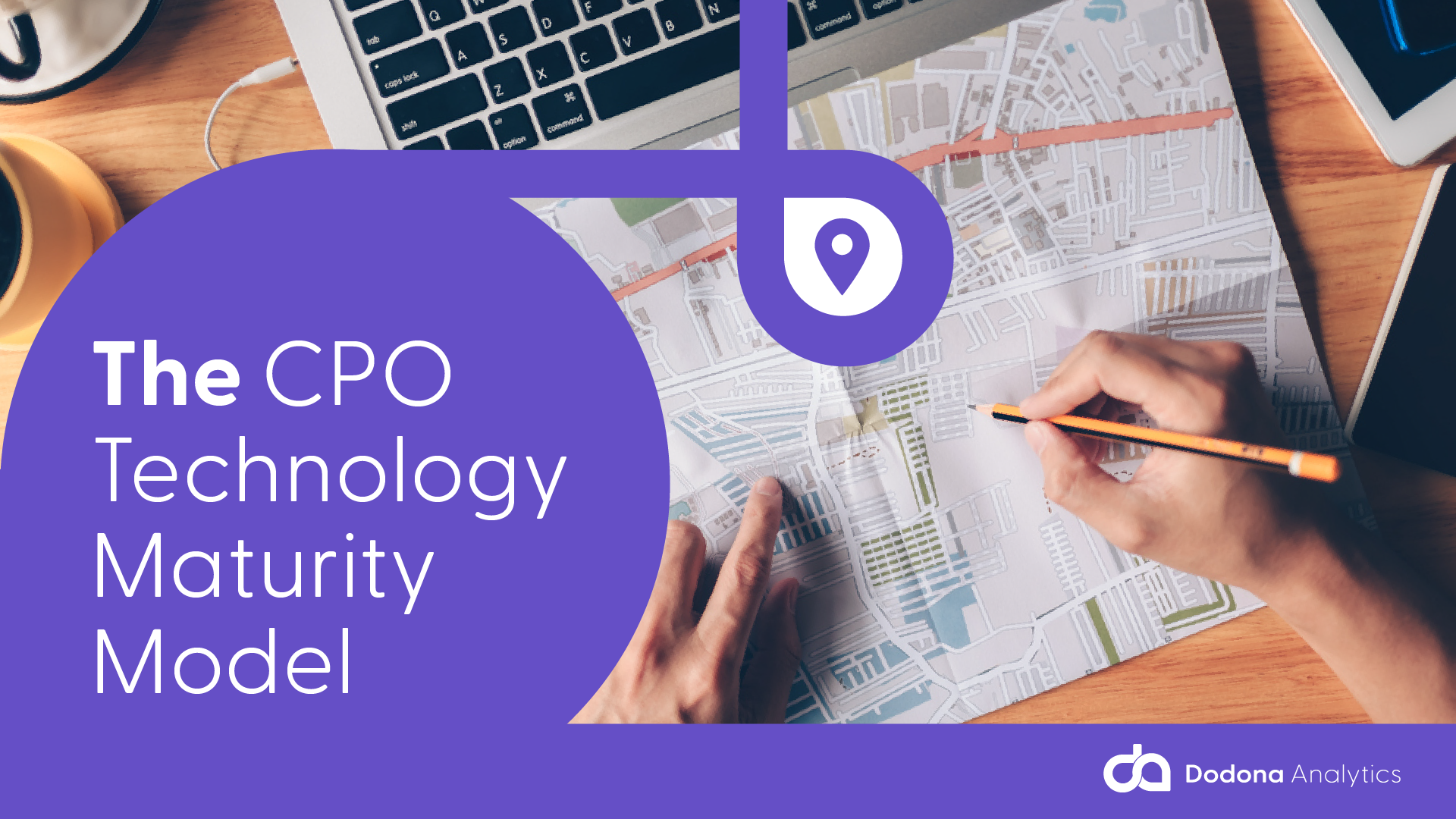
In this post, our co-founder, Chris Chamberlain, shares some of his experience working with successful Charge Point Operators and how technology is a key element to that success.
When building a successful business, charge point operators (CPOs) face two key challenges: the network’s accuracy, finding viable and feasible sites, and scalability in rolling out fast enough to be profitable and meet consumer needs.
A recent European Automobile Manufacturers’ Association report revealed that the EU needs 8 times more charge points per year if it plans to meet its environmental goals by 2030. Whilst accuracy is improving, a significant factor in meeting the demand that I see impacting the CPO’s ability to scale is their approach to site selection.
I have seen CPOs take various approaches to the challenges of accuracy and scale, and the industry is evolving from “gut feel” to a fully-fledged data science approach.
Site selection approaches
Gut feel
Smaller operators starting in a local area often rely on intuition or existing local knowledge. They rely on this knowledge to find spots with high traffic, parking spots near amenities, or locations frequented by existing or potential EV drivers. While this approach can sometimes yield successful outcomes, it overall lacks both accuracy and the ability to scale. It is based on subjective experience instead of data and can overlook factors such as population density and traffic patterns, therefore often resulting in inaccuracy. In terms of scalability, it is impractical to use this approach as the network grows, especially once it crosses into areas unknown to the operators.
Tabs and spreadsheets
A slightly more systemized approach might include Excel, Google Maps, and 20+ browser tabs. This means finding and cross-referencing data, compiling it in a spreadsheet, and using tools such as Excel to perform analyses and pinpoint sites. This approach is time-consuming and overwhelming, while the results are not significantly more optimized. The amount of data makes it easy to overlook key factors and the manual aspect makes it difficult to apply the process to select a large number of charge points.
BI or GIS tools
Another approach uses Business Intelligence (BI) or Geographic Information Systems (GIS) tools. This is a step up for Municipalities and CPOs as they can visualize and analyze spatial data, employing different layers and producing insights invisible to spreadsheets. With the right configuration and data this can lead to a far more accurate and scalable network. However, these tools can be complex and require a steep learning curve, especially for users who are not familiar with geospatial analysis or data analytics. This complexity can make it challenging for operators to fully leverage the capabilities of these tools without adequate expertise, as it is a challenge to extract the relevant information and translate it into useful insights. The approach is also heavily dependent on data, which needs to be of an appropriate level of quality, integrated, updated, and maintained. We have seen very large companies using data sets that are often incomplete and often several years old! Conversely, we have seen people using ‘straight-line projections’ for EV adoption that really have little value from a Data Science perspective.
Build your own
The third choice is to build their own models, upgrading all the existing possibilities by integrating other tools and dedicating a data science and project team to the cause. This approach is high in accuracy and ability to scale and is appropriate for those who have over 18 months of time to build the model and start using it to produce results and endless resources to fund the building and maintenance of such a tool. Four years ago when we first started doing this there were very few commercial options available for site assessment or network planning but now there are a handful of good Data Science companies providing Commercial off the Shelf options. IN short this approach takes time (lost opportunity cost) and can be VERY expensive, where the final cost is unknown at the point of commissioning of the project!
EV Charging Optimization Platform
Alternatively, charge point operators can leverage existing platforms specialized in site selection (like ours!).
There is no need to compile overwhelming sets of data, to have data analysis expertise or a project management team to keep your model running.
The Dodona eMobility EV Charging Optimization platform is an existing solution that aggregates and analyzes vast amounts of data, providing CPOs with comprehensive insights and prioritized lists of potential locations. This approach offers a quicker and more cost-effective solution as it eliminates the need for in-house data analysis and model development while providing the most accurate and scalable process out of all potential approaches.
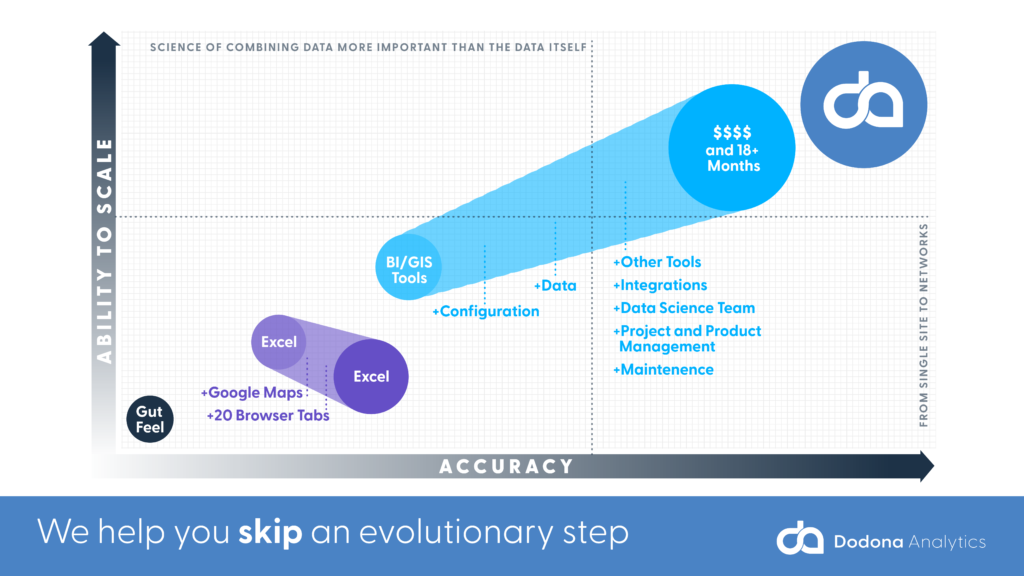
The typical CPO tech evolution
There are typically 3 stages in a CPO tech evolution:
- Startup: In the early stages of their journey, organisations often rely on gut feeling and intuition for site selection. With limited resources and experience, they may initially overlook the need for systematic processes and data-driven approaches. Each decision is made independently, often by different people, lacking consistency and standardized procedures across the business. However, as the business grows, they begin to realize the limitations of relying solely on intuition and recognize the need for more structured processes. This might lead them to dabble in the Tabs and spreadsheets approach as they try to gain more clarity.
- Scaling: As they mature and become more accurate in their site selection, they encounter the need to scale. This might lead them to explore BI or GIS solutions. BI tools provide CPOs with advanced analytics capabilities, while GIS tools offer spatial analysis functionalities. This marks a transition to a data-driven approach.
- Turn to experts: Despite the strengths of the previous approach organisations evolve to reach a point where BI and GIS tools no longer meet their needs. They may seek to develop custom models but soon realize the challenges associated with building and maintaining their own solutions, including the need for specialized data science expertise and ongoing data management, and the cost of development and maintenance. This realization leads organisations to reconsider their approach and explore alternative solutions. They recognize the value of working with experts who already have existing platforms and domain knowledge in the field of electric vehicle infrastructure. By collaborating with established platform providers, they can leverage their expertise, access advanced technologies, and benefit from continuous updates and improvements without the burden of developing and managing their own solutions.
I’ve been doing this for awhile now, and our platform helps organisations skip these expensive and painful evolutionary steps and make strategic data-driven site selection decisions from the very beginning, maximizing network efficiency and minimizing risk.
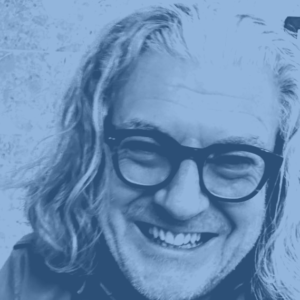
Chris is our co-founder and VP of Strategic Accounts and new markets. He is an evangelist for eMobility and is passionate about helping the sector make better, evidence-based decisions with data science and AI.
You can follow Chris Chamberlain on LinkedIn